What is Machine Learning? Types of Machine Learning? 7 Steps of Machine Learning
In this quick post, we will go through below topics related to Machine Learning:
1. What is Machine Learning?
2. Types of Machine Learning?
3. 7 Steps of Machine Learning
What is Machine Learning?
Machine learning is a subset of artificial intelligence that deals with the design and development of algorithms that can learn from and make predictions on data. These algorithms are used to automatically detect patterns in data and then make predictions or decisions based on those patterns.
Types of Machine Learning?
There are three main types of machine learning: supervised learning, unsupervised learning, and reinforcement learning.
Supervised learning
Supervised learning is where the data is labeled and the algorithm is told what to learn. For example, a supervised learning algorithm might be used to classify images of animals as either cats or dogs.
Unsupervised learning
Unsupervised learning is where the data is not labeled and the algorithm is left to find structure in the data on its own. For example, an unsupervised learning algorithm might be used to cluster data points into different groups.
Reinforcement learning
Reinforcement learning is where the algorithm is given feedback on its predictions and learns from its mistakes. For example, a reinforcement learning algorithm might be used to play a game such as chess.
7 Steps of Machine Learning
2. Data Exploration and Visualization: This is the process of exploring the data to look for patterns and trends. This may involve using various data visualization techniques to help see the data in different ways.
3. Model Selection and Training: This is the process of choosing a machine learning model and training it on the data. This step can be challenging, as there are many different types of models to choose from, and it is often not clear which one will work best for a given problem.
4. Model Evaluation: This is the process of assessing how well the model performs on data that it has not seen before. This is important in order to gauge how well the model will generalize to new data.
5. Model Tuning: This is the process of adjusting the model to improve its performance on the data. This may involve changing the model architecture, the training data, or the hyperparameters.
6. Deployment: This is the process of putting the model into production, so that it can be used by others. This may involve packaging the model into a web service or app, or deploying it on a server.
7. Maintenance: This is the process of keeping the model up-to-date and making sure it continues to perform well. This may involve retraining the model on new data, or making changes to the model architecture.
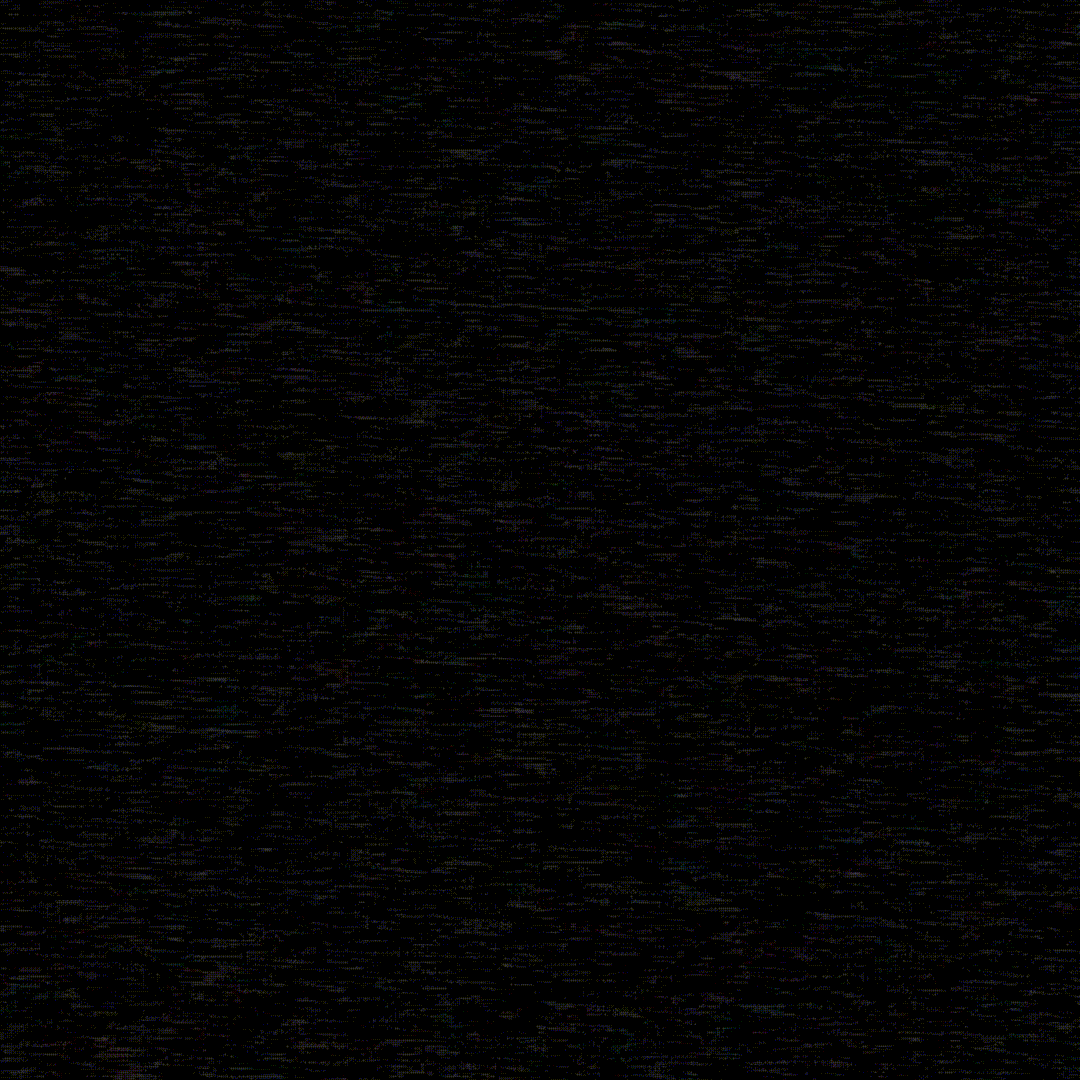
Comments