How to Become a Data Scientist (Step-by-Step) - Process & Road-Map
Becoming a data scientist is a process that involves learning a variety of skills. In this quick post I am going to share Data Science Road-Map, Data Scient Process and Tools & Techniques which will help you to achieve your dream role as Data Scientist.
1. The Data Science Process (Step by Step):
2. Data Science Road Map:
The Data Science Process (Step by Step):
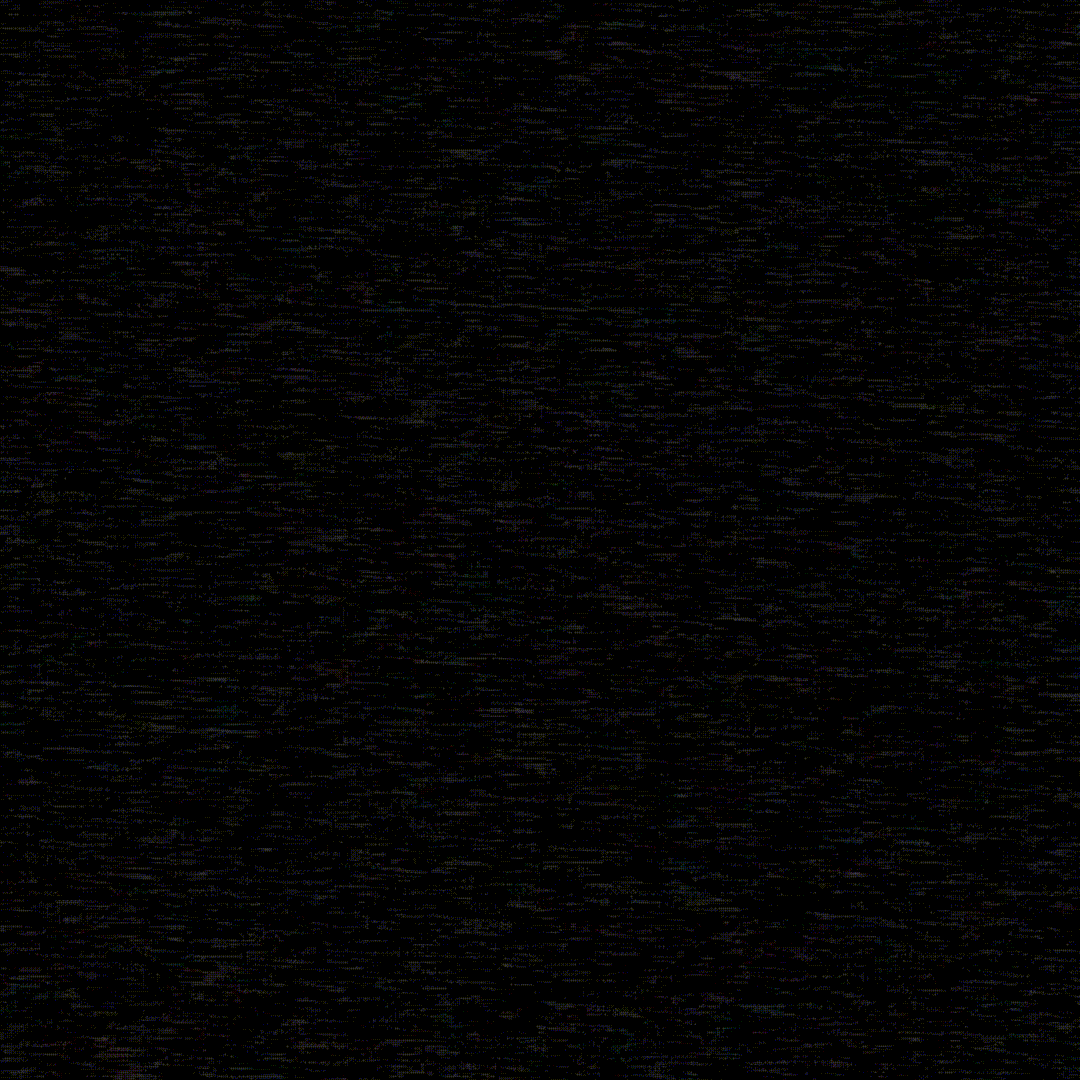
The data science process is the series of steps that data scientists use to solve problems. It includes everything from data collection and cleaning to data analysis and modeling. This process is a great way to learn about the different steps involved in data science.
1. Determine what kind of data scientist you want to be.
There are many different types of data scientists, each with their own unique skillset. Before you begin your journey to becoming a data scientist, it's important to decide which type of data scientist you want to be. Do you want to be a machine learning engineer? A big data analyst? A business intelligence expert? Once you've decided on the type of data scientist you want to be, you can begin to focus on acquiring the skills and knowledge necessary to excel in that field.
2. Choose the right tools and programming languages.
There are many different tools and programming languages used in data science. Some of the most popular include R, Python, and SQL. It's important to choose the right tools and programming languages for the job at hand. If you're working with large datasets, for example, you'll want to use a language that is efficient at handling big data. If you're working on a machine learning project, you'll want to use a language that has robust machine learning libraries.
3. Understand the math and statistics behind data science.
Data science is a highly mathematical and statistical field. In order to be successful, it's important to have a strong understanding of the math and statistics behind data science. This includes topics such as linear algebra, calculus, and probability.
4. Get comfortable with working with data.
One of the most important skills for a data scientist is comfort with working with data. This means being able to clean data, wrangle data, and perform exploratory data analysis. If you're not comfortable working with data, it will be difficult to succeed as a data scientist.
5. Build your own data science portfolio.
A data science portfolio is a great way to show off your skills and experience to potential employers. It can also be a valuable tool for learning and keeping up with the latest data science trends. Building a data science portfolio is a great way to make yourself stand out from the crowd.
Comments